Digital disruption is a transformation in business models caused by emerging digital technologies. These new technologies can impact the value of existing products and services, thus disrupting the current market and creating a need to reevaluate business operations. Digital disruption is impacting call center operations by transforming the way in which they communicate with customers and market to them.
Call centers can embrace digital disruption and even plan for it by recognizing the signs in their industry, allowing them to get ahead of their competitors. This capability prevents digital disruption from eroding a call center’s previous successes by providing it with new opportunities for growth. Digital disruption in a call center is usually the result of changes in customer needs and responses to fill those needs, helping to keep existing customers happy and finding opportunities to attract new customers.
Impact on Call Centers
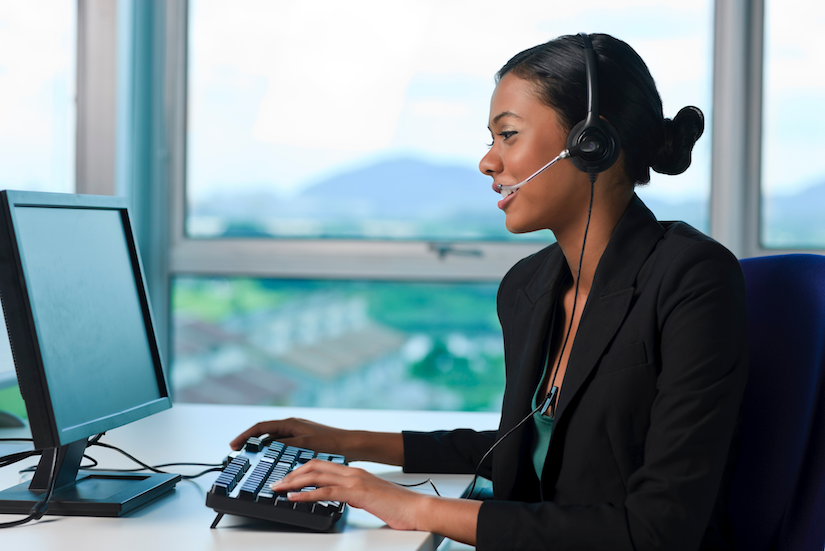
The COVID-19 pandemic resulted in the adoption of new technology as customers accelerated their shift towards online interaction. Customers now make a large percentage of their purchases online and order them delivered, rather than buying products in a brick-and-mortar retail store. This trend was already well underway before the pandemic, but the sudden increase in online activity is exposing gaps in online purchasing processes. These deficiencies illustrate the need to quickly improve the customer experience (CX) for digital customers by obtaining real-time consumer insights into customer behavior.
Changes in Customer Behavior
A recent Medallia Zingle study reports that 77 percent of consumers will now ascertain the degree of personal interaction required before visiting a retail outlet. Furthermore, 87 percent of the respondents in that survey also say retailers should continue to offer options for delivery and curbside pickup. These results help explain why curbside retail and e-commerce sales have skyrocketed, while overall retail sales have declined. A recent report from eMarketer shows ecommerce increased 18 percent in retail.
These results aren’t restricted to the retail sector, as financial services are currently experiencing rapid growth as well. Customers are now managing processes such as loan and credit card applications online, which they used to do in person. CNBC reports that mobile banking traffic increased by 85 percent in April 2020, near the beginning of the pandemic in the US. Consumer sectors have generally increased by 15 to 40 percent since then, according to a McKinsey survey from May 2021.
Post-Disruption Technology Trends
Technology is currently blurring the line between physical and digital reality, providing emerging businesses with the opportunity to break existing monopolies. The always-on environment of today’s commerce is fiercely competitive, resulting in the need to “innovate or die” for all businesses. A high degree of flexibility is now required to stay ahead of the competition.
The changes in consumer behavior resulting from technological advances requires a corresponding change in the way brands present their products and services. This overall trend affects business operations as well as communications with customers, especially marketing. Specific trends in marketing technology include chatbots, data practices and artificial intelligence (AI), all of which have the potential to fundamentally change business practices for companies that can innovate in these areas.
Chatbots
Chatbots were in widespread use by 2018, although most customers still found them frustrating to work with. Nevertheless, 40 percent of large businesses had adopted chatbots to some extent by 2019. Since then, however, technological advances have greatly improved their ability to process natural language. Today’s chatbots accomplish this by analyzing user sentiment and other characteristics of human speech that provide contextual clues to the speaker’s intent.
They still have a ways to go, but chatbots are in the process of revolutionizing the customer service industry. They can now perform many tasks that previously required direct human interaction, including job recruiting, loans and news reporting. This trend will save businesses time by streamlining CX and will be one of the most disruptive technologies in this area for the near future.
Data Practices
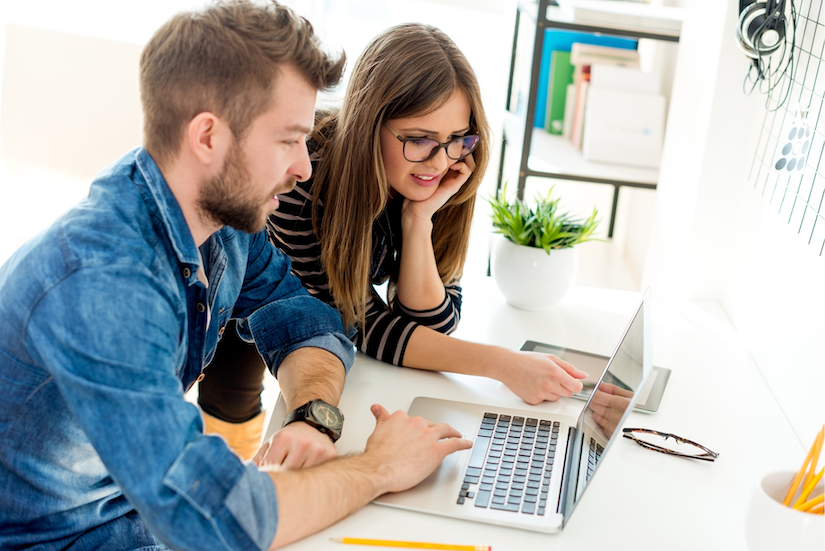
The analysis of data is now essential for remaining competitive in business. Data analytics allows businesses to obtain useful insights into customer behavior, allowing them to make good decisions in developing new products and services. This capability is forming the basis of innovation for all businesses, but it has also created some challenges. The biggest problems with modern data collection generally relate to the massive amount of data that organizations have and not knowing what to do
with that data.
Humans have created 90 percent of all the data in the world within the last two years, but we’re only using one percent of it effectively. The effort expended in collecting this data is wasted if that data is never used, making data analytics vital to remaining competitive. Leaders in data-driven business analytics currently include Amazon, IBM, Microsoft, Salesforce and SAP. The widespread use of cloud platforms allows organizations to get more use from their data by investing in analytics.
AI
AI includes any technology that can perform tasks that would otherwise require a human. Machine learning (ML) is a branch of AI in which the technology imitates human learning processes. ML allows technology to process data and adjust its behavior based on those results, all with no human intervention.
This feature helps businesses deal with their abundance of data, resulting in more useful insights and faster innovation. ML is changing the way we perform, share and interpret analytics by making data-driven connections, a process commonly known as augmented analytics (AA). The potential of ML for relieving humans of the need to perform these tedious tasks will help organizations focus on their long-term strategies for innovation rather than mundane data processing.
Stakes
The migration of customers to online shopping provides businesses with a greater opportunity to obtain real-time insights into their behavior. This process is vital for monitoring consumer response to digital offerings, especially for those accustomed to making purchases in physical stores. These customers are more likely to abandon shopping carts because they find online shopping to be difficult.
This problem is especially common when shopping for clothing online. Consumers can typically browse through many options for style, size and color but still have trouble finding exactly what they want, even when using the many filters that e-commerce sites typically provide. Missing images and nonfunctioning product links are typical reasons for consumers to find another place to shop, and it’s much easier to switch websites than to drive to a physical store.
Three-fourths of consumers experimented with changes in their shopping behavior during the pandemic, according to the 2021 McKinsey survey. Availability, value and convenience were the driving factors for customers who tried new brands, resulting in a greater tendency to contact a business when they’re unable to solve the problems online. Zendesk reports that customer service calls reached a record high in May 2021, which is typically a much more expensive channel than self-service options. Increased competition in e-commerce means that businesses must work harder to increase the efficiency of customer service needed to maintain customer loyalty. Real-time insights are highly important in reaching this goal.
Options
Organizations must obtain clarity on the reasons for customer behavior, whether it’s inadequate online support, poor content or a broken workflow. This information is vital for delivering the best experience across all consumer segments. The shift to online shopping means that digital channels are now the primary means of communication between businesses and customers.
Learning about customer behavior requires a thorough review of the website, its applications and all other digital touch points. Important information to discover during this process include whether customers can find the information they need on the website and its ease of navigation. It’s also critical to identify the content that can improve the customers’ experience and any aspects of the business’s digital presence that’s frustrating for them.
An organization’s digital transformation requires the ability to collect information from multiple sources, integrate it into a single repository and analyze it. Real-time insights are required to make effective decisions in a rapidly changing market driven by customer demand. These insights are essential for creating innovations in the customer’s journey towards a purchase, which will typically disrupt methodologies based on traditional market research. Marketers must therefore become more agile when generating consumer insights by using more dynamic approaches to research.
Use of Real-time Data
Technology has now reached the point where marketers can use real-time data in a way that helps customers, thus generating profit for businesses. This capability is a far cry from the traditional approach to marketing based on previous purchases of similar products.
Assume for this example that Jane is a representative customer who is married with children and an affluent homeowner. She typically shops at her favorite retailer, both online and in a physical store. Her purchases are based on her previous purchases as well as those of customers with profiles similar to hers. Jane also considers the latest styles when making a purchase, which happen to be yoga pants on this occasion.
Once Jane makes her purchase, that’s usually the end of the retailer’s interaction with her with the possible exception of a generic follow-up email. Imagine instead that the retailer sends Jane an email with a health theme based on her purchase of the yoga pants. Jane clicks the link in the email, which plays a video about raising healthy children. She then receives a text message advising her of a 15 percent one-day discount on workout equipment if she buys it through the store’s mobile app.
Jane has never bought these items from this retailer before, but she takes advantage of the offer and buys a new gym bag. This journey began with the simple task of buying yoga pants, but the greater degree of customer engagement resulted in a second purchase. This data-activated marketing technique is based on the consumer’s real-time needs and behavior, which represents a key part of the current growth in e-commerce. It can increase total sales by up to 20 percent, and even more for online sales.
Furthermore, this strategy can significantly improve the return on investment (ROI) for marketing across all channels, including mobile apps and websites. Virtual reality (VR) experiences through products such as headsets and cars will also become effective marketing channels in the near future.
Businesses routinely test the impact of different customer experiences on purchases, but they typically do so with the purchases in isolation from each other. Attempting to scale this process quickly presents the challenge of determining which aspects of customer behavior to prioritize. Referring back to Jane, should marketers primarily target her as a yoga enthusiast or a mother? In some cases, marketers may also decide that Jane is part of a new segment that combines both of these attributes.
The challenge of prioritizing segments has continued to plague marketers, despite the development of solutions like customer relationship management (CRM), marketing resource management (MRM) and master data management (MDM). All of these solutions help consolidate data sources, manage segmentation and organize workflow for the purpose of improving customer relationships. They rely on traditional methods such as campaigns and list pulls, but none of them take full advantage of this data to obtain insights into customer behavior. Obtaining the adaptive modeling and agile data utilization needed to scale personalized interactions with customers requires a new approach.
Customer Data Platform
The Customer Data Platform (CDP) helps users make discoveries about data, and automates the decision-making process by allowing marketers to scale customer interactions based on data in real time. CDP is slowly becoming an industry standard, and third-party platforms are currently shaping the online marketing space. McKinsey reports that using a CDP successfully requires the following four steps whether it’s to incorporate the CDP into an existing system or starting from scratch.
1. Data Foundation
Businesses often have a relatively complete view of their customers, although this information typically resides in multiple discrete quotations. These data silos prevent organizations from connecting data in a way that allows them to use it effectively. CDP uses information that the company already has but combines it to create a meaningful customer profile that’s accessible across the organization.
The process of “feeding” a CDP begins by combining as much of the available data as possible and continuing to build on it over time. The goal is to achieve customer profiles that create value, which requires advanced analytics to process the data and ML to obtain insights. A CDP generates increasingly granular subsegments of customers over time based on events such as a purchase, site visit or social media post expressing interest in a product. These events help expand the CDP’s data set, allowing the organization to respond to customers in real time and develop new ways of engaging them. In addition, the insights obtained from this process should extend beyond the customer’s specific response to a campaign, typically by targeting product development towards particular segments.
Many well-known companies struggle to fully understand customers who make infrequent purchases. This process generally involves combining CRM data with consumer data from social media to build models that help identify prospects most likely to buy products in a particular category. For example, turning display ads on Facebook on to increase customer targeting can increase ROI by up to 100 percent compared to an average Facebook audience. Mapping third-party data to segments with a data management platform (DMP) can enhance the CX for both known and unknown customers, provided that data exists. This process can improve conversion and engagement as measured by acquisition value (AV), lifetime value (LTV) and net promoter score (NPS).
2. Decisioning
The decisioning function of the CDP allows marketers to identify the best content for a particular customer and channel. This step also scores customers according to their potential value, and a set of business rules then matches those scores with specific offers and prioritizes the offer to send. ML is increasingly likely to perform the matching process, allowing companies to greatly improve their engagement with customers by developing more personalized messages based on customer behavior. Companies that are more sophisticated in the use of the CDP can build a decisioning model that functions across all of their distribution channels. This benefit requires advanced analytics and modeling techniques to assess the impact of one channel on another, as customers routinely change channels along their decision journey.
This behavior can be easy to determine such as abandoned carts or browsing without purchasing, but they can also be more nuanced like activity based on time of day and segment. These customer behaviors thus become triggers that evoke actions on the part of the business. A CDP’s decision engine then develops a set of triggers and their desired outcomes based on the company’s responses. The companies that are more sophisticated in the use of the CDP can build a decisioning model that functions across all of their distribution channels. This benefit requires sophisticated analytics and modeling techniques to assess the impact of one channel on another, as customers routinely change channels along their decision journey.
Effective decisioning requires repeated testing to validate hypotheses and predict outcomes. This process should become increasingly sophisticated over time as subsequent models build upon previous ones. Assume for this example that a company is testing offers for different customer groups according to factors such as age, location, previous purchases and customers who have viewed a particular website within a specified period of time. This process may seem complex but a decisioning engine can easily prioritize offers with the best return rate for each customer group. This capability allows the business to scale its test results without the risk of conflicting offers or inconsistent CX.
3. Design
Designing offers that customers will find attractive requires businesses to understand their behavior, engage them and deliver the selected offer with appropriate content. The primary challenge in performing this process successfully is that the departments within the company often operate independently, especially in large enterprises. The owners of each channel tend to test customers and engage them on that channel exclusively. However, the real benefits of CDP only occur when an organization begins using cross-functional teams composed of members from multiple departments such as IT, legal, marketing and merchandising, each of which may focus on a specific aspect of the customer journey.
These teams should establish a clear set of priorities and assign the members responsible for delivering on them. Cross-functional teams are also effective at designing new hypotheses for engaging customers and devising experiments to identify the best offer for a particular segment. Analytics assist with this process by evaluating opportunities, testing the impact of offers and obtaining insights from those tests. Team members can then tag the content that will deliver each offer, which is often associated with a trigger. This step allows the company to send an offer as soon as it’s needed. The design phase of CDP thus allows organizations to greatly reduce their testing period and increase testing volume.
4. Distribution
A CDP distribution system is the delivery pipeline for the content intended for the end user, which could be an ad server, content management platform (CMP) or demand side platform (DSP). Distribution systems can be indiscriminate by simply blasting out emails to a mailing list with little customization. However, a CDP engine can greatly increase the focus of a marketing instrument by using triggers and tagged content. Sending specific messages to each sub-segment across all channels is typically a much more efficient use of effort.
Organizations often develop a library of application programming interfaces (API) that help the CDP tie into the marketing technologies used to deliver and track CX. This integration creates a feedback loop that sends information on customer engagement, response and conversion back to the CDP.
Implementing a Data-Activation Framework
The effectiveness of data-activation efforts vary greatly, so a case-driven approach is usually the best way to rank tests by opportunity. This process evaluates the impact of each case on customer behavior, while also considering the effort needed to implement that case. Deploying a CDP doesn’t replace existing customer data systems, unlike a complete digital transformation. Instead, a CDP merely provides an operational solution that an organization can integrate into its systems with relative ease. Marketers usually find that they already have many of the marketing and technology requirements for a CDP, although they may not be using them properly.
Conclusion
Real-time insights into customer behavior can greatly increase your market agility. This post-disruption technology also provides many opportunities to grow in a competitive marketplace through innovation.
Aceyus can help you begin mapping your customers’ journey with our software that analyzes customer data in real time. Download our eBook, “A Contact Center’s Guide to: Customer Journey Mapping and Improving the Customer Experience” to learn how you can provide customers with a better overall experience by pinpointing opportunities for growth and understanding the buyer’s emotions and goals.